Interview with the CEO of Footprint Analytics
Q1: Navy, can you give us an overview of what Footprint Analytics is currently working on?
Footprint Analytics is dedicated to building a structured data platform that bridges the gap between Web2 and Web3 data.
We specialize in structuring data. Despite Web3’s relative advantage over Web2 in transparent on-chain data, some challenges remain. These include the nascent state of the industry, the lack of standardized practices, and the lack of organized data. As a result, data application becomes problematic.
As an example, consider the scenario where you want to access transaction data on Opensea from multiple chains such as ethereum, solana, and Polygon. This process involves understanding OpenSea’s business model, examining the smart contract code, and sequentially extracting transaction data from each chain.
This process is complex. First of all, it is complex and prone to errors throughout the data collection process. Second, it is technically complex, given the differences in ledger design and data structures between chains. Finally, it leads to waste of resources. In a scenario where 1000 people need this data, they will have to go through a similar complex process 1000 times. This significant duplication significantly hinders data collection efficiency and wastes computing resources.
This brings us to the purpose of Footprint Analytics: to abstract data from different industries such as GameFi, NFTs, and DeFi and create standardized data applications for the Web3 industry. This will allow developers and industry participants to access and analyze data efficiently and accurately.
To date, we have launched platforms on more than 20 blockchains, organized into three key segments:
As a data platform, Footprint is a natural fit for AI. Artificial intelligence encompasses three key aspects: computing power, data, and algorithms. Among them, computing power is the foundation that underpins AI model training and application. At the same time, data is the core of AI, and algorithms determine AI performance, including model accuracy and application effectiveness.
Of these, data is undoubtedly the most important and indispensable. Data is the lifeblood of industries and projects, and its importance extends to core areas such as privacy and compliance, where its value is immeasurable. The data may be unaffordable given its entanglement in privacy and compliance issues. AI acts as both a consumer and producer of data.
Currently, Footprint’s application of combining data and AI covers several key aspects:
The contribution of artificial intelligence to our platform during the data content creation phase is critical. Initially, we use artificial intelligence to create data processing code to provide users with a smoother data analysis experience.
More specifically, we direct innovation in two specific directions.
First, we select and categorize the reference data. Taking recently deployed contracts on the blockchain as an example, our AI can independently determine the protocol to which a contract belongs, the type of contract, and even whether the contract falls into categories such as LP or Swap on Dex platforms. This intelligent structuring and classification greatly increases data accessibility.
Second, we can generate higher-level domain data based on our reference data. For example, GameFi, NFT, etc. We use artificial intelligence to generate data in fields and provide users with richer data sources. This approach improves the quality of data content and enables users to better understand data across different industries.
We introduced an AI-based smart analytics function to improve front-end user experience. As mentioned above, users experience a conversation-like experience with ChatGPT when they use Footprint for data analysis. Users can ask questions and receive relevant data analysis reports instantly. The underlying logic involves translating text into SQL queries, which significantly lowers the barrier of entry for data analysis.
Finally, when it comes to user support, we developed an artificial intelligence-powered customer service bot. To create a custom AI customer service bot for Footprint, we feed the AI with data from Footprint covering GameFi, NFT, DeFi, and other spaces. This AI includes data types, data definitions, API usage, etc. Provided immediate assistance to users by answering questions about using Footprint, including: This has greatly increased the efficiency of customer support while reducing the amount of manual work.
However, it is worth noting that although artificial intelligence applications can increase productivity and help solve many challenges, they may not be omniscient. Based on our data processing experience, AI can help solve approximately 70% to 80% of challenges.
Q3: What challenges are likely to arise in integrating AI with Web3? Are there issues with technical complexity, user experience, intellectual property compliance, or ethical considerations?
From a broader perspective, regardless of the domain in which AI is applied, a critical consideration is the level of acceptance of AI’s fault tolerance. Different application scenarios have different fault tolerance requirements. There is a need to balance the accuracy and reliability of artificial intelligence with humans’ tolerance for errors.
For example, the decision to trust AI or a doctor in healthcare may involve challenges related to trust. In the investment field, AI can provide factors that influence the direction of BTC prices, but people may still have doubts when making actual buying or selling decisions.
However, precise accuracy may not be very important in marketing and operational analysis such as user profiling and tiering, as small errors will not significantly affect it. As a result, fault tolerance is more readily accepted in these contexts.
Currently, Footprint is focusing primarily on data in its efforts to integrate AI with Web3, which presents its own challenges:
First, the first challenge is data generation; In particular, it provides high-quality data for AI to achieve more efficient and accurate data generation capabilities. This relationship between AI and data can be compared to the engine and fuel of a car; Here, AI is the engine and data is the fuel. No matter how advanced the engine is, lack of quality fuel will prevent optimum performance.
This raises the question of how to generate high-quality data, for example, how to generate data quickly and automatically in areas such as GameFi, NFTs, DeFi and others. This involves automatically organizing data connections, essentially creating a data graph. More specifically, it involves identifying factors such as protocols with which contracts are associated, contract types, providers, and other relevant details. The main purpose of this process is to continuously provide high-quality data to increase the efficiency and accuracy of artificial intelligence in data generation, thus creating a virtuous cycle.
The second challenge is data privacy. While Web3 is fundamentally committed to decentralization and transparency, the need for privacy may become paramount as the industry evolves. This includes protecting users’ identities, assets, and transaction information. This poses a dilemma: The transparency of data on the blockchain is decreasing, limiting the amount of data that AI can access. However, as the industry progresses, this problem will be solved and homomorphic cryptography will be a possible solution.
As a result, the convergence of AI and Web3 is inherently intertwined with a fundamental issue: data accessibility. At its core, the biggest challenge for AI lies in accessing high-quality data.
Q4: Although artificial intelligence is not a new concept, the convergence of artificial intelligence and Web3 is still in its infancy. So, Navy, what potential areas or combinations of AI in Web3 do you believe could serve as a breakthrough that would attract significant user influx to Web3 and facilitate mass adoption?
I believe that achieving significant integration and adoption of Web3 and AI depends on overcoming two key challenges. First, there is a need to provide advanced services to Web3 creators and developers, especially in areas such as GameFi, NFTs, and social platforms. Second, it is imperative to reduce implementation barriers to enable smoother user entry into the Web3 environment.
Let’s start serving the developer community. Two main types of applications came to the fore in this field.
One category is AI-powered development platforms. These platforms use AI technology to automate the creation of code templates. Whether for building DEX platforms or NFT marketplaces, these platforms can significantly increase development efficiency by intelligently generating code templates tailored to developers’ specific needs.
Artificial intelligence in games can speed up the creation of game models and rendering of visuals, thus speeding up the game development and launch process. These platforms have allowed developers to focus more on creativity and innovation rather than spending excessive time on repetitive basic tasks.
The other category revolves around AI-powered data platforms. These platforms use AI to independently generate domain-specific data across a variety of verticals such as GameFi, NFTs, SocialFi, and DeFi. The goal is to lower the threshold for developers to use and apply data and to simplify data analysis and usage.
Thanks to AI, these platforms can automatically generate diverse datasets, enrich developers with rich data sources, and improve their understanding of market trends, user behavior, and more. By providing comprehensive data support to developers, these data platforms eliminate barriers to data use and accelerate the emergence of creative applications.
Mass adoption has always been a key challenge in the Web3 space. For example, the market has recently seen the emergence of blockchain solutions aimed at increasing transactions per second (TPS) with almost negligible fees. Additionally, solutions such as the MPC wallet overcome migration challenges, effectively removing the main barrier to migrating from Web2 to Web3.
The solution to these challenges does not depend solely on artificial intelligence technology; It is intertwined with the holistic evolution and development of the Web3 ecosystem. While AI plays a key role in improving efficiency and reducing barriers, the underlying infrastructure and growth of Web3 remain key factors in solving the mass adoption problem.
Contact Navy Tse
#Interview #CEO #Footprint #Analytics
Q1: Navy, can you give us an overview of what Footprint Analytics is currently working on?
Footprint Analytics is dedicated to building a structured data platform that bridges the gap between Web2 and Web3 data.
We specialize in structuring data. Despite Web3’s relative advantage over Web2 in transparent on-chain data, some challenges remain. These include the nascent state of the industry, the lack of standardized practices, and the lack of organized data. As a result, data application becomes problematic.
As an example, consider the scenario where you want to access transaction data on Opensea from multiple chains such as ethereum, solana, and Polygon. This process involves understanding OpenSea’s business model, examining the smart contract code, and sequentially extracting transaction data from each chain.
This process is complex. First of all, it is complex and prone to errors throughout the data collection process. Second, it is technically complex, given the differences in ledger design and data structures between chains. Finally, it leads to waste of resources. In a scenario where 1000 people need this data, they will have to go through a similar complex process 1000 times. This significant duplication significantly hinders data collection efficiency and wastes computing resources.
This brings us to the purpose of Footprint Analytics: to abstract data from different industries such as GameFi, NFTs, and DeFi and create standardized data applications for the Web3 industry. This will allow developers and industry participants to access and analyze data efficiently and accurately.
To date, we have launched platforms on more than 20 blockchains, organized into three key segments:
- Footprint Growth Analysis as an Industry Solution: Similar to the Web3 version of Google Analytics, dedicated solutions for Web3 projects in marketing growth and operational analytics guide projects towards data-driven growth.
- Zero-Code Data Analysis Tools: Offering a similar experience to ChatGPT, this tool allows users to get data analysis reports through simple queries and responses. In the foreseeable future, the use of on-chain data will be greatly simplified; There will be no need for a complex understanding of Web3 business logic or advanced programming skills, making the transition from Web2 to Web3 easier.
- Free Unified API: Through a unified multi-chain and cross-chain API, this feature facilitates cross-chain data access across multiple chains, providing users with a seamless experience of retrieving data from multiple chains at no cost.
As a data platform, Footprint is a natural fit for AI. Artificial intelligence encompasses three key aspects: computing power, data, and algorithms. Among them, computing power is the foundation that underpins AI model training and application. At the same time, data is the core of AI, and algorithms determine AI performance, including model accuracy and application effectiveness.
Of these, data is undoubtedly the most important and indispensable. Data is the lifeblood of industries and projects, and its importance extends to core areas such as privacy and compliance, where its value is immeasurable. The data may be unaffordable given its entanglement in privacy and compliance issues. AI acts as both a consumer and producer of data.
Currently, Footprint’s application of combining data and AI covers several key aspects:
The contribution of artificial intelligence to our platform during the data content creation phase is critical. Initially, we use artificial intelligence to create data processing code to provide users with a smoother data analysis experience.
More specifically, we direct innovation in two specific directions.
First, we select and categorize the reference data. Taking recently deployed contracts on the blockchain as an example, our AI can independently determine the protocol to which a contract belongs, the type of contract, and even whether the contract falls into categories such as LP or Swap on Dex platforms. This intelligent structuring and classification greatly increases data accessibility.
Second, we can generate higher-level domain data based on our reference data. For example, GameFi, NFT, etc. We use artificial intelligence to generate data in fields and provide users with richer data sources. This approach improves the quality of data content and enables users to better understand data across different industries.
We introduced an AI-based smart analytics function to improve front-end user experience. As mentioned above, users experience a conversation-like experience with ChatGPT when they use Footprint for data analysis. Users can ask questions and receive relevant data analysis reports instantly. The underlying logic involves translating text into SQL queries, which significantly lowers the barrier of entry for data analysis.
Finally, when it comes to user support, we developed an artificial intelligence-powered customer service bot. To create a custom AI customer service bot for Footprint, we feed the AI with data from Footprint covering GameFi, NFT, DeFi, and other spaces. This AI includes data types, data definitions, API usage, etc. Provided immediate assistance to users by answering questions about using Footprint, including: This has greatly increased the efficiency of customer support while reducing the amount of manual work.
However, it is worth noting that although artificial intelligence applications can increase productivity and help solve many challenges, they may not be omniscient. Based on our data processing experience, AI can help solve approximately 70% to 80% of challenges.
Q3: What challenges are likely to arise in integrating AI with Web3? Are there issues with technical complexity, user experience, intellectual property compliance, or ethical considerations?
From a broader perspective, regardless of the domain in which AI is applied, a critical consideration is the level of acceptance of AI’s fault tolerance. Different application scenarios have different fault tolerance requirements. There is a need to balance the accuracy and reliability of artificial intelligence with humans’ tolerance for errors.
For example, the decision to trust AI or a doctor in healthcare may involve challenges related to trust. In the investment field, AI can provide factors that influence the direction of BTC prices, but people may still have doubts when making actual buying or selling decisions.
However, precise accuracy may not be very important in marketing and operational analysis such as user profiling and tiering, as small errors will not significantly affect it. As a result, fault tolerance is more readily accepted in these contexts.
Currently, Footprint is focusing primarily on data in its efforts to integrate AI with Web3, which presents its own challenges:
First, the first challenge is data generation; In particular, it provides high-quality data for AI to achieve more efficient and accurate data generation capabilities. This relationship between AI and data can be compared to the engine and fuel of a car; Here, AI is the engine and data is the fuel. No matter how advanced the engine is, lack of quality fuel will prevent optimum performance.
This raises the question of how to generate high-quality data, for example, how to generate data quickly and automatically in areas such as GameFi, NFTs, DeFi and others. This involves automatically organizing data connections, essentially creating a data graph. More specifically, it involves identifying factors such as protocols with which contracts are associated, contract types, providers, and other relevant details. The main purpose of this process is to continuously provide high-quality data to increase the efficiency and accuracy of artificial intelligence in data generation, thus creating a virtuous cycle.
The second challenge is data privacy. While Web3 is fundamentally committed to decentralization and transparency, the need for privacy may become paramount as the industry evolves. This includes protecting users’ identities, assets, and transaction information. This poses a dilemma: The transparency of data on the blockchain is decreasing, limiting the amount of data that AI can access. However, as the industry progresses, this problem will be solved and homomorphic cryptography will be a possible solution.
As a result, the convergence of AI and Web3 is inherently intertwined with a fundamental issue: data accessibility. At its core, the biggest challenge for AI lies in accessing high-quality data.
Q4: Although artificial intelligence is not a new concept, the convergence of artificial intelligence and Web3 is still in its infancy. So, Navy, what potential areas or combinations of AI in Web3 do you believe could serve as a breakthrough that would attract significant user influx to Web3 and facilitate mass adoption?
I believe that achieving significant integration and adoption of Web3 and AI depends on overcoming two key challenges. First, there is a need to provide advanced services to Web3 creators and developers, especially in areas such as GameFi, NFTs, and social platforms. Second, it is imperative to reduce implementation barriers to enable smoother user entry into the Web3 environment.
Let’s start serving the developer community. Two main types of applications came to the fore in this field.
One category is AI-powered development platforms. These platforms use AI technology to automate the creation of code templates. Whether for building DEX platforms or NFT marketplaces, these platforms can significantly increase development efficiency by intelligently generating code templates tailored to developers’ specific needs.
Artificial intelligence in games can speed up the creation of game models and rendering of visuals, thus speeding up the game development and launch process. These platforms have allowed developers to focus more on creativity and innovation rather than spending excessive time on repetitive basic tasks.
The other category revolves around AI-powered data platforms. These platforms use AI to independently generate domain-specific data across a variety of verticals such as GameFi, NFTs, SocialFi, and DeFi. The goal is to lower the threshold for developers to use and apply data and to simplify data analysis and usage.
Thanks to AI, these platforms can automatically generate diverse datasets, enrich developers with rich data sources, and improve their understanding of market trends, user behavior, and more. By providing comprehensive data support to developers, these data platforms eliminate barriers to data use and accelerate the emergence of creative applications.
Mass adoption has always been a key challenge in the Web3 space. For example, the market has recently seen the emergence of blockchain solutions aimed at increasing transactions per second (TPS) with almost negligible fees. Additionally, solutions such as the MPC wallet overcome migration challenges, effectively removing the main barrier to migrating from Web2 to Web3.
The solution to these challenges does not depend solely on artificial intelligence technology; It is intertwined with the holistic evolution and development of the Web3 ecosystem. While AI plays a key role in improving efficiency and reducing barriers, the underlying infrastructure and growth of Web3 remain key factors in solving the mass adoption problem.
Contact Navy Tse
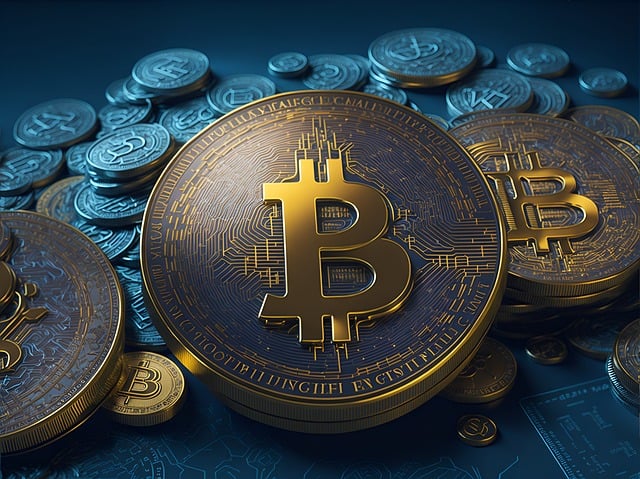
#Interview #CEO #Footprint #Analytics